Beyond Basic TAR: Three Ways to Maximize Review Efficiency Utilizing Technology
Beyond Basic TAR: Three Ways to Maximize Review Efficiency Utilizing Technology
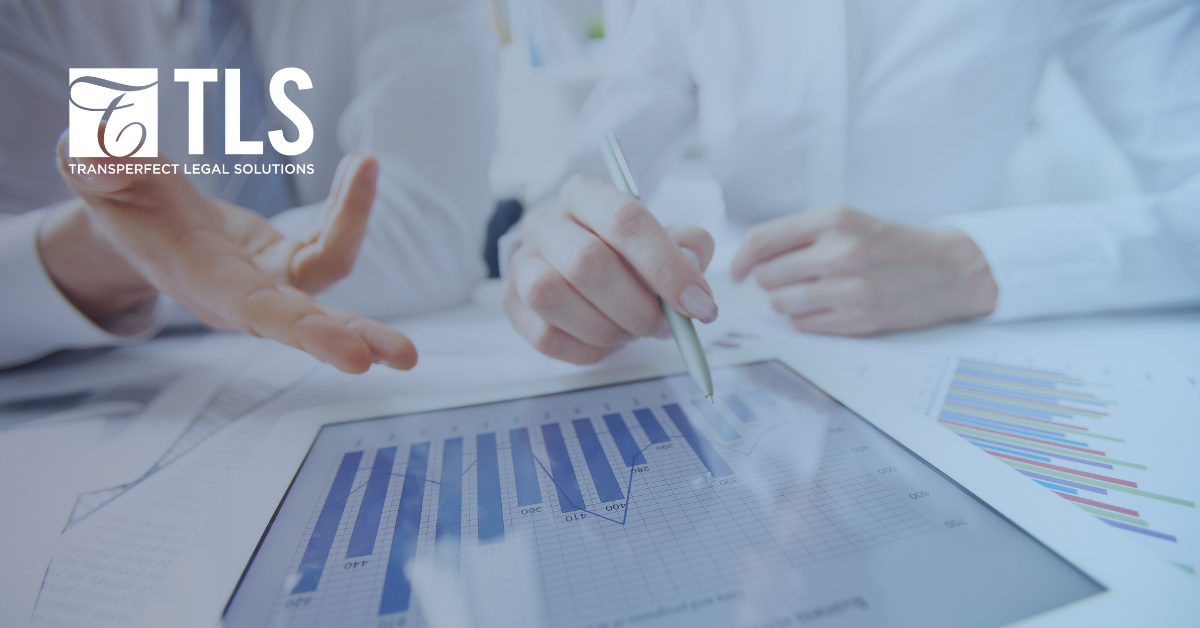
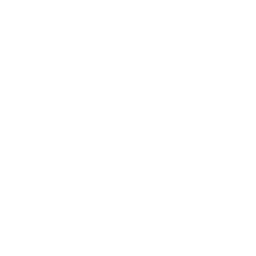
Technology-assisted review (TAR) comes in many flavors and can help significantly decrease the amount of manual review required for a matter. That said, not all TAR tools are created equal. Standard TAR workflows can be significantly enhanced by thinking outside the box, increasing a model’s efficiency, decreasing the number of documents needing manual review, enhancing review consistency, and moreover, decreasing costs. Here we examine three such techniques along with associated case studies that have proved significant efficiencies in review.
Using Pre-Review and Advanced Analytics Techniques to Increase Richness and Decrease the Size of Your TAR Control Set
TAR 1.0 is a predictive coding technique in which a relatively small number of training documents are used to categorize documents in a binary decision, most typically as “relevant” or “not relevant.” These documents are compared against a benchmark, also known as a control set, in order to determine a model’s efficacy. This is one of the most time consuming and costly portions of a TAR 1.0 review, as the best practice is for these documents to be reviewed by one to three subject matter experts (SMEs). A control set by definition must have an adequate number of positive and negative examples to meet a statistical confidence and margin of error. Often, it is difficult to find an adequate number of positive examples as data sets with low richness (richness is a metric of how many positive examples exist in the dataset) are common, especially in TAR 1.0 projects where most protocols require search terms not be used to cull the data. In lieu of search terms, other culling mechanisms may be used with proper sign-off by the involved parties, thereby increasing data set richness and decreasing the size of your control set. By removing a large amount of non-relevant data, there’s a greater probability of finding relevant documents when randomly sampling data.
Pre-review and advanced analytics are tools used in analyzing data sets in order to get better insight to make bulk decisions. These tools vary wildly from platform to platform, but here are a few of the most commonly used in the context of TAR 1.0 pre-culling:
- Sent Domain Analysis
Analyzing emails from a sent domain can be extremely instructive in culling patently non-relevant data. For example, if a large portion of data is coming from @nytimes.com, such as daily news articles, these may be completely unrelated to the issues at hand and can be confidently removed from the data set prior to TAR.
- File Type Restrictions
When collecting electronic documents, there will often be file types that do not contain user-generated content, such as log files and system files. These files should be analyzed by the team and excluded from TAR where appropriate.
- Clustering
Clustering is a tool where the analytics engine will self-organize data based on co-occurrence of concepts. Although we would rarely suggest removing data based on a cluster (and if so, we would strongly suggest reviewing a statistically significant sample of the data), it can be useful by means of concepts, terms, or metadata that can be targeted as potentially non-relevant. For example, if a cluster contains concepts related to contracts, but the case is not concerning a contractual dispute, these concepts or terms may be used to further exclude non-relevant data.
- Search Term Analysis
Although regulatory agencies typically don’t allow search terms to be run prior to TAR 1.0, there are certain protocols that allow for it. By not only running search terms but also analyzing high hit count terms, the amount of data ultimately pushed to TAR will be reduced and richness will be increased.
Using TAR to QC Coding Consistency for Faster, More Accurate Results
In a TAR 2.0/continuous active learning (CAL) workflow, documents are scored based on conceptual similarity to previously manually coded documents. As these scores are based on the total number of manually coded documents, and CAL is most often run by contract review teams, there are frequently inconsistent manual coding decisions between reviewers that affect a document’s score. Because we analyze the relevancy rates at each score to determine a suitable review cutoff point, any inconsistency—especially in the lower ranked bands—can affect the decision as to which score should ultimately cut off review. By implementing strong quality control (QC) mechanisms at the lower ranked documents (e.g., having a strong reviewer QC low ranked documents that are marked relevant), the inconsistencies at these ranks are reduced, which ultimately increases the cut-off score and significantly reduces the number of documents required for review.
Case Example: Our client was issued a supplemental information request by the Canadian Competition Bureau related to an intended merger and recruited TLS to assist with the expedient collection of 760,000 documents from 13 custodians. The time frame for review and production was limited to three weeks. The initial universe of documents collected was quickly culled down to 586,000 using threading to focus on the most inclusive iterations of the document threads. SMEs reviewed several small influential and diverse training sets to determine initial rankings prior to engaging the contract review team. The team then began reviewing the documents based on the initial rankings, and TAR 2.0 learning was applied each evening following completion of the day’s review.
In the above case, we found that documents with very low initial scores had a significant number of documents coded as relevant by the review team. QC of the low-ranking, relevant documents confirmed that a substantial portion of these were incorrectly coded and in fact non-relevant. A handful of QC reviewers were then tasked with focusing on low-ranking documents categorized as relevant to update coding where applicable. In doing so, the relevance rate at lower rankings dropped significantly. In this matter, by focusing QC on just under 5,000 low-ranking documents, contemporaneous with review and TAR 2.0, the team was able to comfortably meet its deadline by culling approximately 50,000 low ranking documents that would have otherwise required time consuming review.
Switching TAR Horses Mid-Stream to Maximize Conceptual Analytics Efficiency
TAR analytics engines typically use algorithms that have been in use for decades in other non-legal realms. The most often used are logistic regression, support vector machines, or some amalgamated approach. Each algorithm has pros and cons. That being said, how data is analyzed and filtered into the index also has a large impact on how well an index works against a dataset. For example, certain tools analyze each word separately in creating an index, while others also contain phrase detection. More advanced tools will recognize entities found within a dataset to give further meaning to the concepts they build. This means that regardless of the algorithm, how a dataset is constructed and filtered can have a huge impact on the results of TAR. Here we explore how switching analytics engines can benefit stubborn data sets where TAR appears to reach diminishing returns.
Case Example: Our client partnered with us for the hosting and review of 76,000 documents from 26 custodians to be reviewed by nine contract reviewers. TLS suggested CAL be used over the review population to reduce the time and costs associated with the project. The team then began reviewing the documents based on the machine’s built-in review queue, learning as the review progressed. In order to confirm that the CAL project didn’t miss a significant number of relevant documents, the team proceeded with model validation. The particular validation here is called an “elusion test,” in which a statistical significant sampling of the random documents within the unreviewed data set are reviewed by a SME and the percentage of documents missed or eluded by the tool is estimated. This validation allows the legal team to have defensibility around the process and results of the CAL model. After five weeks and several elusion tests, TLS recommended trying a different CAL technology. After approximately two days of reviewing the newly ranked documents, the elusion rate dropped 73% and the CAL process was considered complete.
In the above matter, even after reviewing a significant portion of the data and most of the documents with very low rankings, the elusion rate was found to be 8.75%, which was higher than the client requirements. The simple CAL tool treated each word on its own within the index and therefore was less meaningful. At this point, we decided to switch to a different tool that had better filtering and indexing technology, specifically with phrase detection, giving the index more meaning. The tool was implemented, and the review team continued with two more days of review to address the newly highly scored documents. A new elusion test was sampled and reviewed, finding only 2.4% of the documents were eluded by the model, a 73% increase in efficiency.