Finding and Understanding Multilingual Documents: Where Technical Nuance & Language Converge
Finding and Understanding Multilingual Documents: Where Technical Nuance & Language Converge
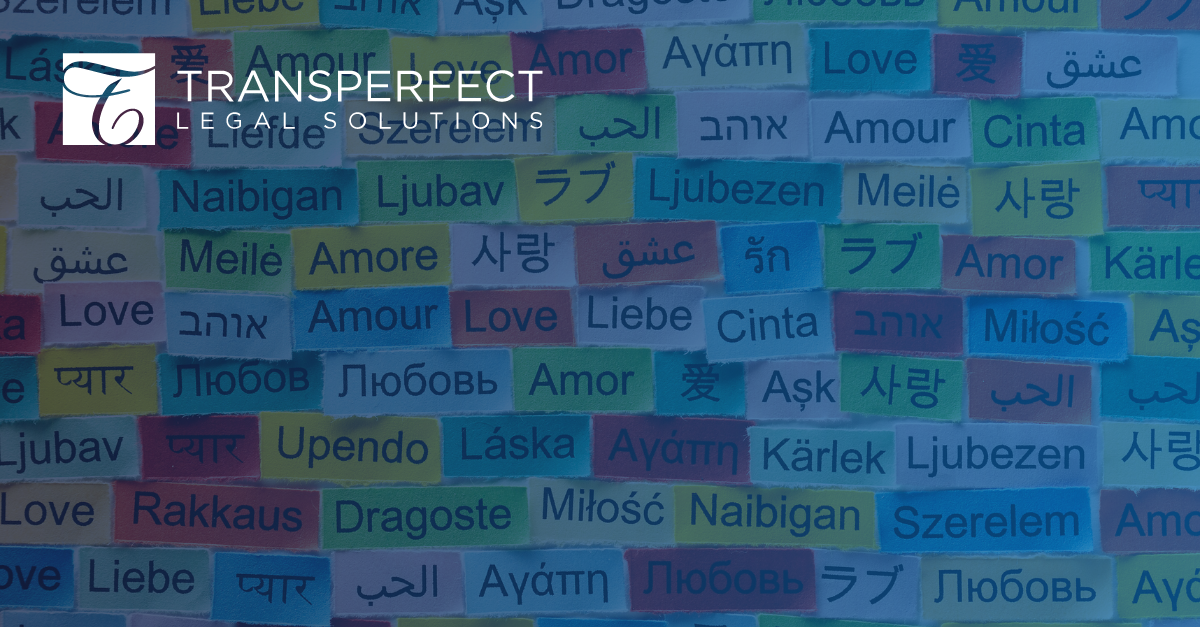
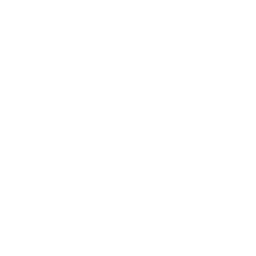
We live in a global environment where commercial disputes, white-collar crime, and antitrust issues frequently cross international borders. There are thousands of non-English e-discovery litigations and investigations every year with billions of non-English documents hidden inside. When multilingual data is involved, every step of the process must pivot to accommodate the nuances and challenges foreign language brings. This requires significant linguistic insight paired with technical know-how considerably beyond the typical skill set of an e-discovery project manager.
The Challenges
Communications and e-docs are central to understanding the factual basis of any matter. Yet, with multilingual data, developing that factual understanding is hindered by the language barrier between the case team and the evidence. This barrier is overcome in various ways—but not all means for bridging said gap are created equal. Further, choosing the right workflows isn’t black and white. Workflow decisions are ultimately governed by cost, speed, and quality considerations, and these considerations must balance one another on the “trade-off triangle”. Multilingual data sets are supported by highly complex services and software—and the best workflows (among many) are often finely balanced. Adding to the complexity, as cases progress, objectives, priorities, and imperatives often shift. The tech needs to as well.
A further, often less appreciated challenge is that most e-discovery software is built in the US with English in mind and optimized accordingly. This is not to say e-discovery technology only handles English, but the vast structural and morphological differences between languages create all sorts of blind spots up and down the EDRM. Being unaware of these blind spots usually means evidence is missed.
Blind Spots Hide in Plain Sight if you Know What you’re Looking at. So, What do They Look Like?
Search Indexes & Non-English Search Term Translations
Take, for example, the proximity search operator, SQL search indexes and the Japanese language.
For any language, the search index contains the text from documents structured and normalized in very particular ways. Indexing facilitates text searching, a process that is governed by search operators. But every language has distinct morphological characteristics, meaning it slots into an index with different governing rules. These differences hold significant implications for search.
Project managers are conversant in the operators governing English, but all languages to varying degrees function differently in search indexes. Take this EN/JP example.
English Term: Toshiba W/10 Sony
Japanese Translation: 東芝 W/10 ソニー
To the untrained eye, the W/10 looks like a perfectly reasonable translated term, yet the Japanese widely misses the mark on two fronts.
JP Term Deficiency1: English v Japanese – Indexing and Search
The first miss is due to a technical point called tokenization. Tokenization is the science of separating masses of text into smaller units such as sentences, words, or sub-words for search.
In other words, all e-discovery platforms tokenize and index English at the word level. That is, “Sony” in English indexes as one word. In Japanese, each character counts as one word. So, ソニー is considered three “words” by the index. In English, the W/10 will serve to find Toshiba & Sony anywhere in the same sentence up to 10 words apart. Whereas, in Japanese, the W/10 operator will only return results with around two to three actual words between the terms.
JP Term Deficiency2: English v Japanese – Morphological Differences
The second front is morphology. Japanese has three main written forms: katakana, kanji, and hiragana. The difference between kanji and katakana is notable because katakana typically contains more characters per word than kanji. In the example, Toshiba is spelled in kanji 東芝, and Sony is spelled in katakana ソニー. Because tokenisation and the search target is likely to contain many katakana words, the distance needs to increase from 10 to 35. When adjusting for these two considerations, 東芝 W/35 ソニー will return results much closer to W/10.
Similarly, on the morphology front, search wildcards in Japanese terms are a red flag. They’re unnecessary due to the absence of pluralization and verb conjugations. If present, wildcards signal potential under-translation of the terms and missing documents.
These are just two examples of search operators in a single language that must shift to achieve the right results. As one navigates the non-English world of search, many similar, nuanced technical points need to be addressed for each language. This is also true from processing to production with enormous effects on review pools and case outcomes.
Machine Translation
Let's look at another example of language bumping into technology found in the review phase—machine translation (MT).
The first impression or the first translation of a document often becomes the attorney’s understanding of that document. If you’ve ever interacted with Asian language MT, you may have encountered occasionally nonsensical output. There are many root causes, and all of them are generally correctable.
When a MT engine fails to adequately carry the message from the source document, the attorney’s understanding of that document may be incorrect, and the evidence could be missed, misinterpreted, or misapplied.
Continuing with the Japanese morphology and tokenization from above, let's look at an example of those factors within a MT engine.
In English, sentences and words are neatly signposted with capitalization, punctuation, and spacing. Japanese, by contrast, contains less sentence signposting and no word signposting. Japanese characters are all words in their own right and often combine to create entirely new words but without the delineation of spacing.
From the earlier search example: 東芝 individually mean ‘east’ 東, and ‘lawn’ 芝, or understood together as Toshiba.
In Japanese, even before a single word translates, MT engines are first deciding on sentence segmentation, followed by word segmentation. With all the weird and wonderful ways humans express themselves in language, correct segmentation is tricky to consistently get perfectly right. Because words in a sentence depend on one another, making one wrong text division easily results in the engine producing nonsense MT where ‘Toshiba’ translates as ‘east lawn’.
There are several other ways ‘東芝 ends up translating as ‘east lawn’ and the frequency of such mistranslations somewhat depend on the language pair and how the engine was trained.
Important to appreciate is that mistranslations are easily fixable, you just need the right language service provider. Not all providers are created equal. In multilingual matters, legal teams need a service provider that understands language as much as they understand e-discovery. Case teams need support from technical and lingual consultants, linguists, and e-discovery professionals well-versed in these nuances. The multilingual know-how arising from this mix of talent is indispensable to a defensible outcome.