Four Techniques to Reduce Ediscovery Burdens by 40%
Four Techniques to Reduce Ediscovery Burdens by 40%
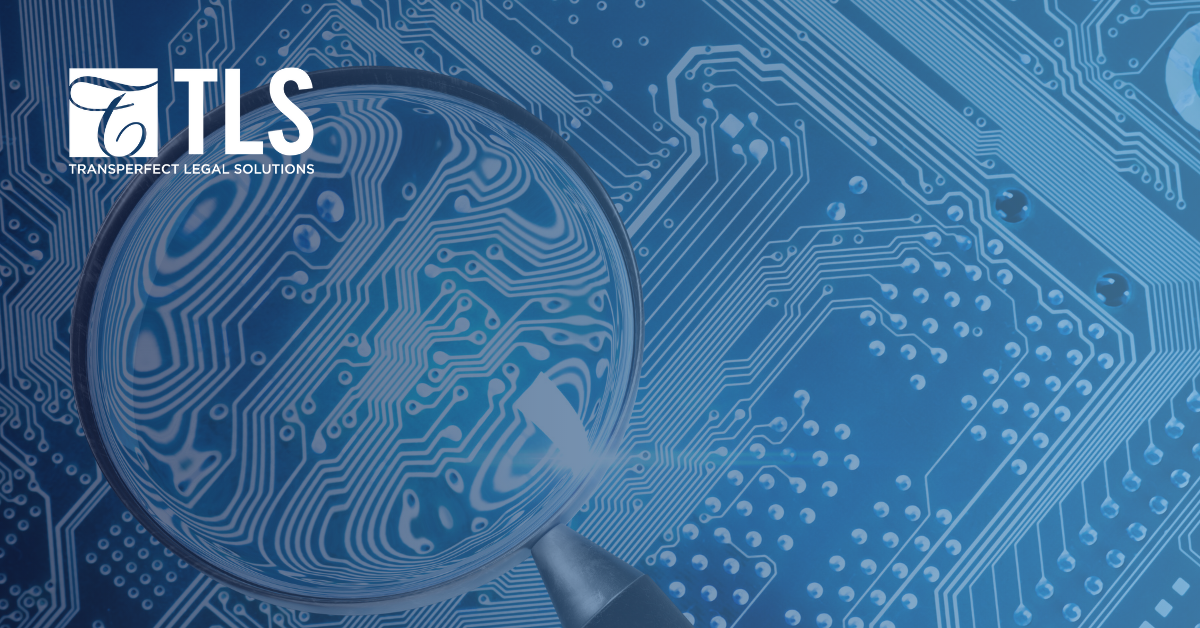
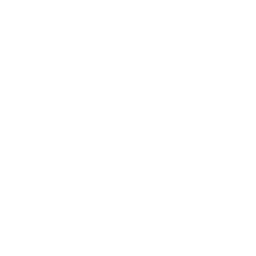
Ediscovery in the Big Data era has become cost-prohibitive to many litigants. Efforts to address this problem through amendments to the rules of civil procedure have been, unsurprisingly, slow. Fortunately, new technologies enable advanced workflows that substantially reduce ediscovery burdens while empowering legal teams to access important documents faster—and lead to more efficient, higher quality legal advice. This article describes specific techniques to reduce your ediscovery spend while also increasing the quality of your ediscovery.
Big Data in Ediscovery
Emails are the worst offender. To give some perspective, a multinational company’s employees can generate over 5.2 million emails every day. And, the average office worker receives 120 daily emails. So if a matter involves ten custodians over three-years, the initial data set will consist of nearly one million emails (plus their attachments).
Text messages, instant messaging, document management systems, and cloud collaboration platforms have become ubiquitous to modern business. Take Slack, for example. Launched just six years ago, Slack is already used at over 600,000 organizations, including 43 of the Fortune 100 companies. Users send over one billion new business messages every week. It’s no wonder these various data systems have exploded the size of the typical business’ digital footprint and, in turn, their ediscovery headaches.
The growth of data volumes has wrought havoc on legal budgets, but brought little benefit. More documents does not mean more “hot” documents. In 2014, Microsoft analyzed its litigations and concluded that on average, approximately 10,544,000 pages of electronic documents are ingested into the ediscovery process. 350,000 of those are reviewed, 87,500 are produced, and only 88 are actually used in the proceeding. That equates to an alarming return on investment—one single document is useful for every 119,818 introduced in the ediscovery process.
The net result of this new reality is that seventy-three cents of every dollar spent on ediscovery is on document review (i.e., humans sorting through the hay to find the needles). In turn, the only way to effectively streamline the modern ediscovery process is to “fight fire with fire” –overcome technology-driven problems with technology-driven solutions.
Four Technology-Driven Workflows to Substantially Reduce Ediscovery Burdens
For years, the filtering process in ediscovery has consisted of deduplication, search terms, date filters, and removing NIST files (i.e., computer-generated files with no human content). This traditional four-step process typically results in an 80% reduction in data volumes, leaving 20% for document review. Supplementing this traditional approach with more advanced culling techniques increases filter rates, on an average, to 91.5%, leaving only 8.5% of the documents for review. The net result is an average savings of over 40%.
1. Filters Based on Email Addresses
Search terms are always overinclusive. Many search term miss-hits include SPAM emails, daily industry newsletters, and internal administrative emails that every custodian receives, but have nothing to do with the underlying dispute and do not deduplicate because of different recipient email addresses. An advanced ediscovery platform can quickly generate a list of every sender email and their volume of hits. Armed with that list, one can quickly and defensibly isolate and remove substantial volumes of non-responsive data. This simple step results in substantial downstream savings.
2. Filters Based on File Types
Similar to email filters, a simple but valuable method to reduce downstream costs is to isolate and remove irrelevant file types. An advanced ediscovery platform can quickly generate a report breaking-down the data set by file type (e.g., Word documents, spreadsheets, audio files, video files, etc.). A file type report empowers the legal team to quickly isolate and remove clearly irrelevant documents (e.g., audio and media files that have no relevance whatsoever to a breach of contract dispute). Because ediscovery costs are often based on data volumes measured in bytes, removing large audio and media files also results in substantial savings.
3. Concept Clustering
Concept clustering is an advanced tool that utilizes a form of artificial intelligence known as natural language processing to categorize and sort documents based on their content. If one thinks of a large document corpus as a bag of mixed jellybeans, concept clustering will put all the popcorn jellybeans in one pile, licorice in another, cotton candy in a third, and so on. These smaller clusters of documents present tremendous opportunity to target key documents at the earliest phase of discovery, but also to separate and remove groupings of totally irrelevant documents that happen to hit on search terms and be within the applicable date range. In this way, concept clustering is the single greatest way to mitigate the unintended consequences of inherently imprecise search terms.
4. Technology-Assisted Review (TAR)
After pre-review filtering options are exhausted, advanced ediscovery technologies are also available to significantly streamline the review process, such as TAR (aka predictive coding). TAR programs look for patterns of language in documents marked responsive and patterns of language in documents marked non-responsive. Thus, after a knowledgeable team member reviews and tags a small sampling of documents as responsive/non-responsive, TAR associates language patterns found within the tagging decisions.
Using TAR 2.0 (aka continuous active learning), an algorithm will then analyze the remaining documents and grade them from 0-100 based on how many patterns of responsiveness are found within the documents. The highest-graded documents (i.e., the ones most likely to be responsive) are batched to human reviewers for manual review. This process repeats itself continuously, with each subsequent grading exercise based on a larger volume of tagging decisions, which further educates the machine learning TAR algorithm.
Eventually, reviewers cease to find responsive documents in batches and, after a statistical validation exercise to ensure defensibility, the review process can be terminated so that not every document undergoes human reviewed. Using the aforementioned Microsoft example, rather than reviewing 350,000 documents to find 87,500 responsive documents, it is likely that using TAR 2.0, only 175,000 total would have needed to be reviewed—reducing by half the burden and expense of document review.